Interpretability and explainability are crucial aspects of data science that play a significant role in building trust, understanding complex models, making informed decisions, and ensuring ethical considerations. Here are several key reasons why interpretability and explainability are important in data science:
- Transparency and Trust: Interpretability and explainability help build transparency by providing insights into how models make predictions or decisions. This transparency fosters trust among stakeholders, including users, customers, regulators, and organizations, ensuring they understand the reasoning behind the results.
- Model Understanding: Interpretability allows data scientists and domain experts to gain a deeper understanding of how models work. It helps identify factors or features that are most influential in making predictions or driving certain outcomes. This understanding enables model refinement, improvement, and identification of potential biases or errors.
- Error Detection and Debugging: Interpretability allows for the detection and debugging of errors or biases in models. By being able to interpret and explain the underlying mechanisms, data scientists can identify issues such as data leakage, overfitting, or incorrect model assumptions, leading to more accurate and reliable results.
- Regulatory and Legal Compliance: Certain domains and industries have legal and regulatory requirements for transparency and accountability in decision-making. Interpretability and explainability can help ensure compliance with these regulations, such as the General Data Protection Regulation (GDPR) in the European Union.
- Ethical Considerations: Interpretability and explainability play a vital role in addressing ethical concerns related to
- Accountability and Compliance: Interpretability and explainability are crucial for holding data scientists and organizations accountable for their models and decisions. When models are interpretable and explainable, it becomes possible to trace the decision-making process and identify the responsible parties in case of errors, biases, or unethical behavior. This accountability is essential for maintaining ethical standards, ensuring compliance with regulations, and addressing potential legal implications.
- User Acceptance and Adoption: Interpretability and explainability are important for user acceptance and adoption of data science models. When users can understand how a model arrived at a particular prediction or recommendation, they are more likely to trust and adopt the model in their decision-making processes. By providing explanations and insights, interpretability and explainability enhance the user experience and facilitate the integration of data science solutions into real-world applications.
- User Acceptance and Adoption: Interpretability and explainability are important for user acceptance and adoption of data science models. When users can understand how a model arrived at a particular prediction or recommendation, they are more likely to trust and adopt the model in their decision-making processes. By providing explanations and insights, interpretability and explainability enhance the user experience and facilitate the integration of data science solutions into real-world applications.
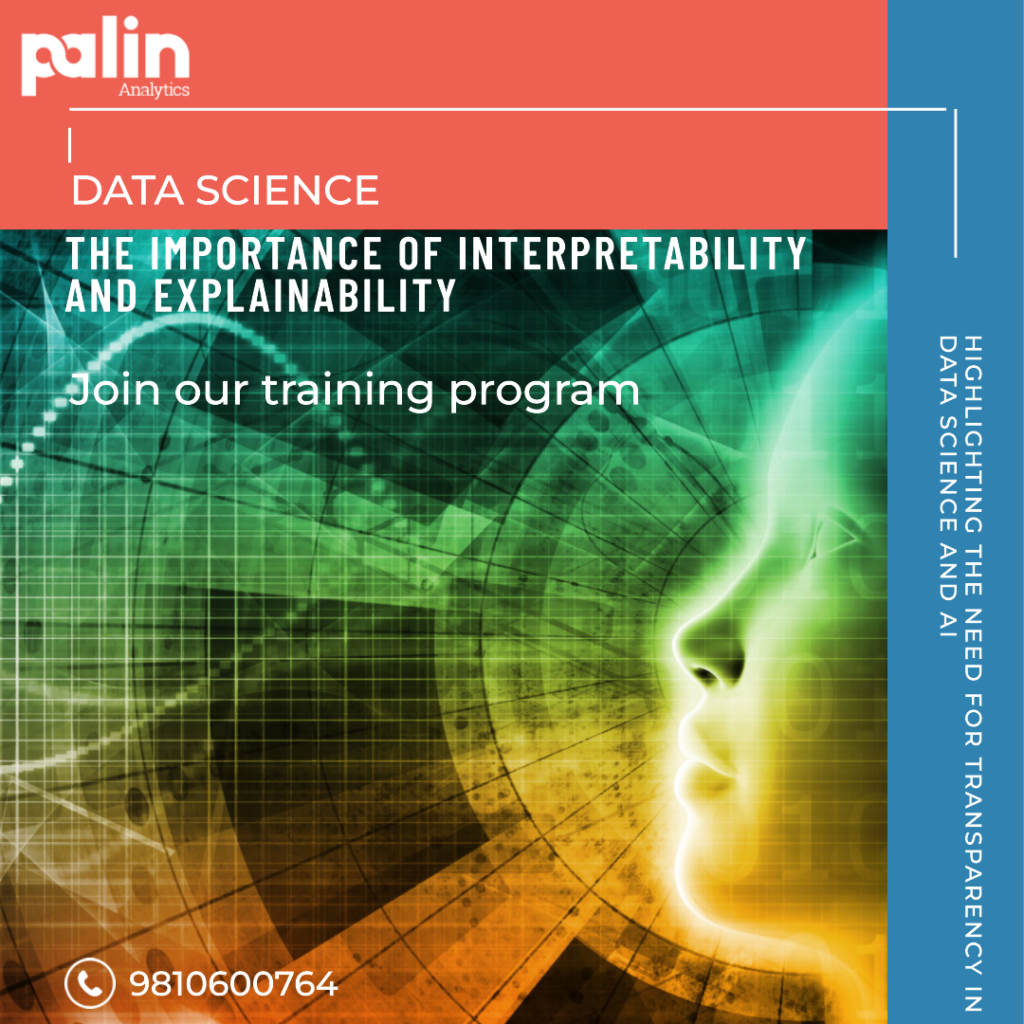