Becoming a data scientist typically requires a combination of education, practical experience, and continuous learning. Here are some steps you can follow to become a data scientist:
- Obtain a solid foundation in mathematics and statistics: Data science involves working with large datasets and performing complex analyses, so a strong understanding of mathematical and statistical concepts is crucial. Take courses or self-study topics such as calculus, linear algebra, probability, and statistics.
- Learn programming languages and tools: Data scientists commonly use programming languages like Python or R for data analysis and manipulation. Familiarize yourself with these languages and their data science libraries, such as NumPy, Pandas, and scikit-learn. Additionally, learn how to work with SQL for database querying and management.
- Earn a degree in a relevant field: While a specific degree in data science is not always necessary, having a background in a related field can be advantageous. Consider pursuing a degree in mathematics, statistics, computer science, engineering, or a related discipline. Some universities now offer specialized data science programs as well.
- Gain practical experience: Hands-on experience is crucial in data science. Start by working on personal projects or participating in Kaggle competitions to gain practical knowledge. Look for internships, research opportunities, or entry-level positions in data-related roles to apply your skills in real-world scenarios.
- Build a portfolio: Create a portfolio of data science projects to showcase your skills and expertise. Include projects that demonstrate your ability to clean, analyze, and visualize data, as well as develop predictive models. Share your portfolio on platforms like GitHub to make it easily accessible to potential employers.
- Continuously learn and stay updated: Data science is a rapidly evolving field, so it’s essential to stay updated with the latest tools, techniques, and research. Follow blogs, attend conferences, participate in online courses, and join data science communities to network with professionals and learn from their experiences.
- Specialize in specific domains or techniques: Data science is a broad field, and specializing in a particular domain or technique can make you stand out. Consider focusing on areas such as machine learning, natural language processing, computer vision, or data visualization, depending on your interests and career goals.
- Pursue advanced education or certifications: While not mandatory, pursuing advanced degrees like a master’s or Ph.D. in data science, statistics, or a related field can provide a deeper understanding of the subject and enhance your career prospects. Additionally, certifications from reputable organizations like Microsoft, Google, or IBM can validate your skills.
- Develop soft skills: In addition to technical skills, data scientists also need strong communication, problem-solving, and critical-thinking abilities. Work on improving your ability to explain complex concepts to non-technical stakeholders and collaborate effectively with multidisciplinary teams.
- Network and seek opportunities: Actively network with professionals in the field, both online and offline. Attend industry events, join data science meetups, and engage in discussions on platforms like LinkedIn or data science forums. Networking can lead to job opportunities, collaborations, and valuable connections.
Remember, becoming a data scientist is a journey that requires dedication and continuous learning. Stay curious, seek out challenges, and never stop expanding your knowledge and skills.
As a data scientist, you can apply your skills and knowledge in various ways. Here are some common applications of data science in different areas:
- Business and Industry: Data science plays a crucial role in businesses and industries, helping them make data-driven decisions and gain insights into their operations. You can work on tasks such as customer segmentation, market analysis, demand forecasting, pricing optimization, and fraud detection.
- Healthcare: Data science is used in healthcare to analyze large volumes of medical data and improve patient outcomes. You can work on projects related to disease prediction, medical imaging analysis, drug discovery, patient risk stratification, and optimizing healthcare resource allocation.
- Finance and Banking: Data science is extensively used in finance and banking for risk assessment, fraud detection, algorithmic trading, credit scoring, customer segmentation, and personalized financial recommendations.
- Marketing and Advertising: Data science helps marketers analyze customer behavior, optimize advertising campaigns, and create personalized marketing strategies. You can work on tasks like sentiment analysis, recommendation systems, customer churn prediction, and social media analytics.
- Internet of Things (IoT): With the increasing use of connected devices, data scientists can analyze IoT data to extract valuable insights. This includes tasks like sensor data analysis, predictive maintenance, smart city planning, and optimizing energy consumption.
- Transportation and Logistics: Data science can be used to optimize transportation routes, predict maintenance needs for vehicles, improve logistics and supply chain operations, and enhance transportation efficiency.
- Social Sciences and Public Policy: Data science techniques are applied in social sciences and public policy to analyze social behavior, predict voting patterns, understand the impact of policies, and develop models for urban planning.
- Environmental Analysis: Data scientists can contribute to environmental research by analyzing data related to climate change, pollution monitoring, wildlife conservation, and sustainable resource management.
- Sports Analytics: Data science is increasingly used in sports to analyze player performance, optimize team strategies, and predict match outcomes. You can work on tasks such as player tracking, performance analysis, injury prediction, and sports betting models.
- Education: Data science can be applied in the education sector to analyze student performance, develop personalized learning models, and improve educational outcomes. You can work on tasks like learning analytics, adaptive learning systems, and intelligent tutoring systems.
These are just a few examples of how data science can be applied in different domains. The field of data science is vast, and you can explore various industries and domains to find areas that align with your interests and expertise.
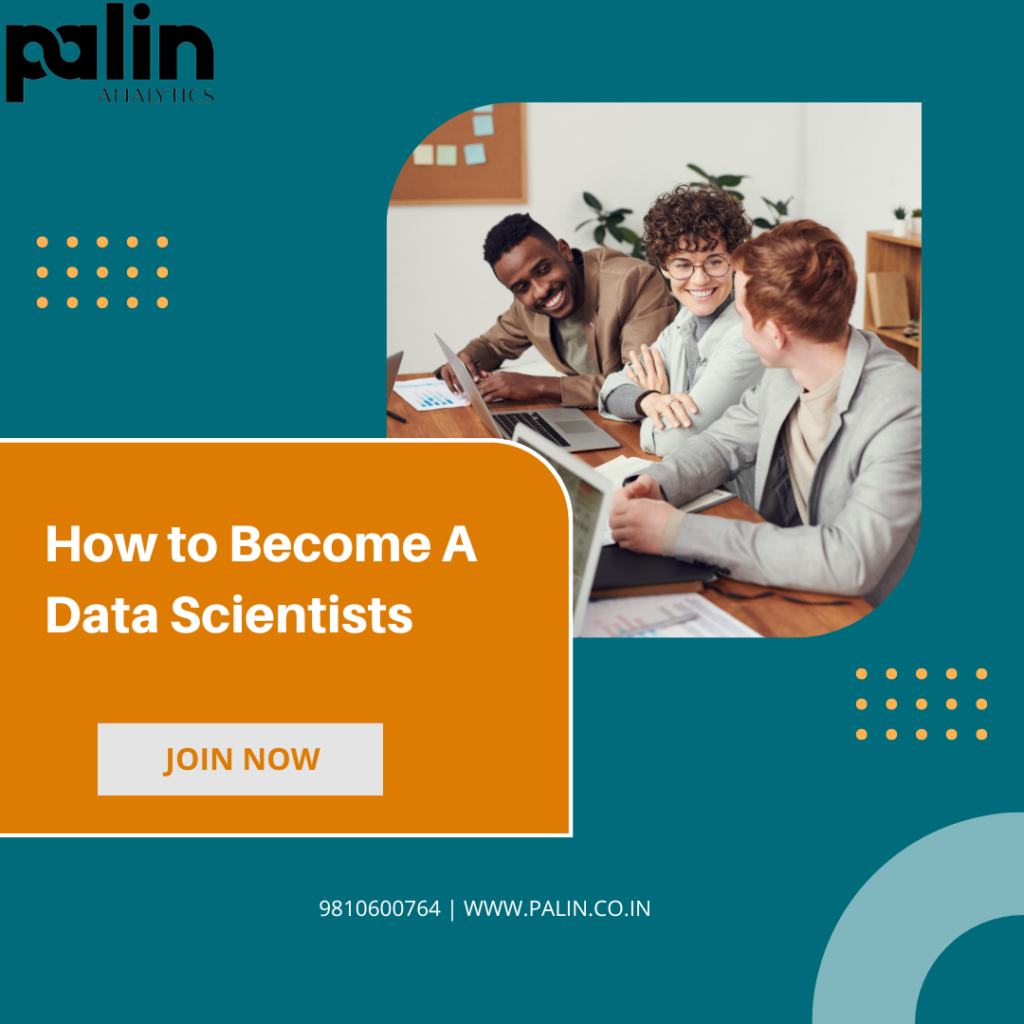